In this article, we will interpret, analyze the COVID-19 DNA sequence data using Biopython.
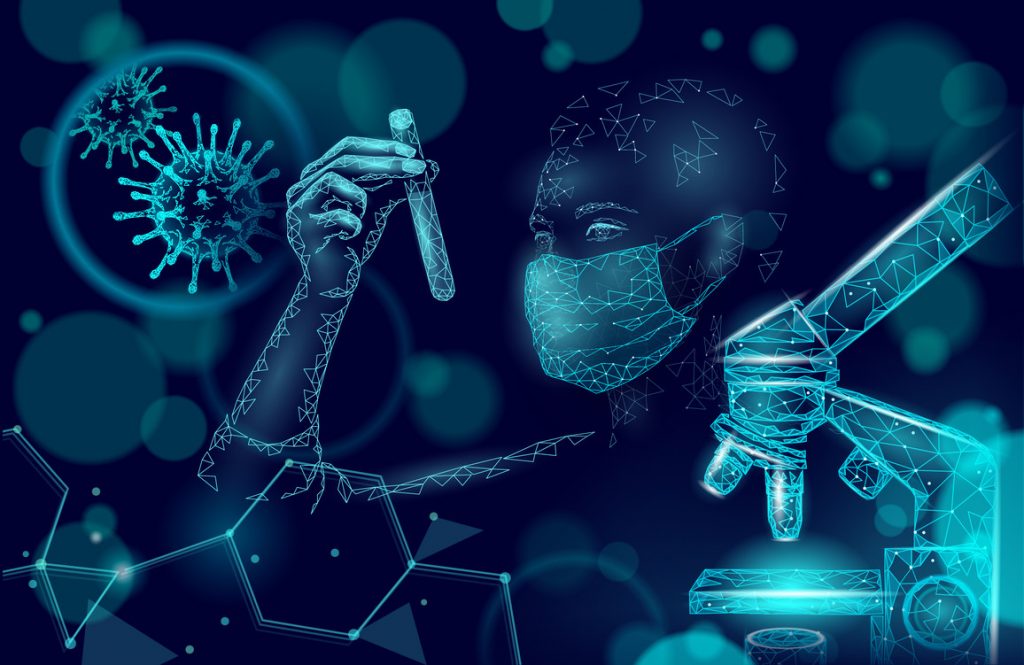
Credits: https://www.bmbf.de/en/coronavirus-status-of-vaccine-development-11210.html
The emerging global infectious COVID-19 coronavirus disease by novel Severe Acute Respiratory Syndrome Coronavirus 2 (SARS-CoV-2) presents critical fulminations to global public health and the economy since it was identified in late December 2019 in China.
Coronaviruses are a large family of viruses that can cause illnesses ranging widely in severity. The first known severe illness caused by a coronavirus appeared with the 2003 Severe Acute Respiratory Syndrome (SARS) epidemic in China. A second outbreak of severe illness originated in 2012 in Saudi Arabia with the Middle East Respiratory Syndrome (MERS). And now the ongoing outbreak of COVID-19.
“By comparing the available genome sequence data for known coronavirus strains, we can firmly determine that COVID-19 originated through natural processes,” said Kristian Andersen, Ph.D., an associate professor of immunology and microbiology at Scripps Research and corresponding author on the paper.
So in this article, we will interpret, analyze the COVID-19 DNA sequence data, and try to get as many insights regarding the proteins that made it up. Later will compare COVID-19 DNA with MERS and SARS and we’ll understand the relationship among them.
The coronaviruses are members of a family of enveloped viruses that replicate in the cytoplasm of animal host cells. They are identified by the presence of a single-stranded plus-sense RNA genome((+)ssRNA classification of viruses) about 30 kb in length that has a 5′ cap structure and 3′ polyadenylation tract. (Larget known virus).
Now let us play with the COVID2–19 DNA sequence data using python.
To start with, install Python packages like Biopython and squiggle will help you when dealing with biological sequence data in Python.
pip install biopython pip install Squiggle
Load the basic libraries:
import numpy as np import pandas as pd pd.plotting.register_matplotlib_converters() import matplotlib.pyplot as plt %matplotlib inline import seaborn as sns import os
The dataset can be downloaded from Kaggle.
We will use Bio.SeqIO from Biopython for parsing DNA sequence data(fasta). It provides a simple uniform interface to input and output assorted sequence file formats.
from Bio import SeqIOfor sequence in SeqIO.parse('/coronavirus/MN908947.fna', "fasta"): print(sequence.seq) print(len(sequence),'nucliotides')
So it produces the sequence and length of the sequence.
GCAATGGATACAACTAGCTACAGAGAAGCTGCTTGTTGTCATCTCGCAAAGGCTCTCAATGACTTCAGTAACTCAGGTTCTGATGTTCTTTACCAACCACCACAAACCTCTATCACCTCAGCTGTTTTGCAGAGTGGTTTTAGAAAAATGGCATTCCCATC......AGAATGACAAAAAAAAAAAAAAAAAAAAAAAAAAAAAAAAA29903 nucliotides
Loading Complementary DNA Sequence into an alignable file
from Bio.SeqRecord import SeqRecord from Bio import SeqIO DNAsequence = SeqIO.read('/coronavirus/MN908947.fna', "fasta")
SeqIO.read() will produce will that basic information regarding the sequence.
SeqRecord(seq=Seq('ATTAAAGGTTTATACCTTCCCAGGTAACAAACCAACCAACTTTCGATCTCTTGT...AAA', SingleLetterAlphabet()), id='MN908947.3', name='MN908947.3', description='MN908947.3 Severe acute respiratory syndrome coronavirus 2 isolate Wuhan-Hu-1, complete genome', dbxrefs=[])
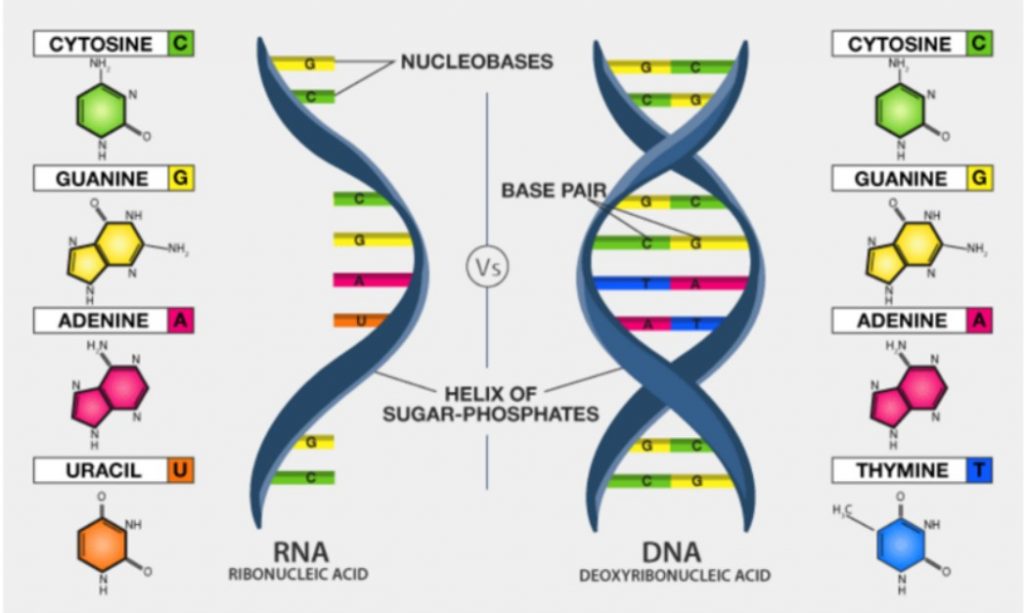
Since input sequence is FASTA (DNA), and Coronavirus is RNA type of virus, we need to:
- Transcribe DNA to RNA (ATTAAAGGTT… => AUUAAAGGUU…)
- Translate RNA to Amino acid sequence (AUUAAAGGUU… => IKGLYLPR*Q…)
In the current scenario, the .fna file starts with ATTAAAGGTT, then we call transcribe() so T (thymine) is replaced with U (uracil), so we get the RNA sequence which starts with AUUAAAGGUU.
DNA = DNAsequence.seq#Convert DNA into mRNA Sequence mRNA = DNA.transcribe() #Transcribe a DNA sequence into RNA. print(mRNA) print('Size : ',len(mRNA))
The transcribe() will convert the DNA to mRNA.
UAUUUUAGUGGAGCAAUGGAUACAACUAGCUACAGAGAAGCUGCUUGUUGUCAUCUCGCAAAGGCUCUCAAUGACUUCAGUAACUCAGGUUC...UAAUAGCUUCUUAGGAGAAUGACAAAAAAAAAAAAAAAAAAAAAAAAAAAAAAAAA Size : 29903
The difference between the DNA and the mRNA is just that the bases T (for Thymine) are replaced with U (for Uracil).
Next, we need to translate the mRNA sequence to amino-acid sequence using translate() method, we get something like IKGLYLPR*Q ( is so-called STOP codon, effectively is a separator for proteins).
Amino_Acid = mRNA.translate(table=1, cds=False) print('Amino Acid', Amino_Acid) print("Length of Protein:",len(Amino_Acid)) print("Length of Original mRNA:",len(mRNA))
In the below output Amino acids are separated by *.
Amino Acid : IKGLYLPR*QTNQLSISCRSVL*TNFKICVAVTRLHA*CTHAV*LITNYCR*QDTSNSSIFCRLLTVSSVLQPIISTSRFRPGVTER*DGEPCPWFQRENTRPTQFACFTGSRRARTWLWRLRGGGLIRGTSTS*RWHLWLSRS*KRRFAST*TALCVHQTFGCSNCTSWSCYG...*SHIAIFNQCVTLGRT*KSHHIFTEATRSTIECTVNNARESCLYGRALMCKINFSSAIPM*F**LLRRMTKKKKKKKKKKLength of Protein : 9967 Length of Original mRNA : 29903
In our scenario, the sequence looks like this: IKGLYLPR*QTNQLSISCRSVL*TNFKICVAVTRLHA, where:
IKGLYLPR encodes the first protein (every letter encodes single amino-acid) QTNQLSISCRSVL encodes the second protein, and so on.
Note that there are fewer sequences in the protein than the mRNA that is because 3 mRNA’s are used to produce a single subunit of a protein, known as an amino acid, using the codon table shown below. The * is used to denote a stop codon, in these regions the protein has finished its full length. Many of these occur frequently and result in short lengths of protein, more likely than not these play a little biological role and will be excluded in further analyses.
Okay, first understand what are Genetic code and DNA codon?
The Genetic code is the set of rules used by living cells to translate information encoded within genetic material (DNA or mRNA sequences of nucleotide triplets, or codons) into proteins.
The standard genetic code is traditionally represented as an RNA codon table because, when proteins are made in a cell by ribosomes, it is mRNA that directs protein synthesis. The mRNA sequence is determined by the sequence of genomic DNA. Here are some features of codons:
- Most codons specify an amino acid
- Three “stop” codons mark the end of a protein
- One “start” codon, AUG, marks the beginning of a protein and also encodes the amino acid methionine.
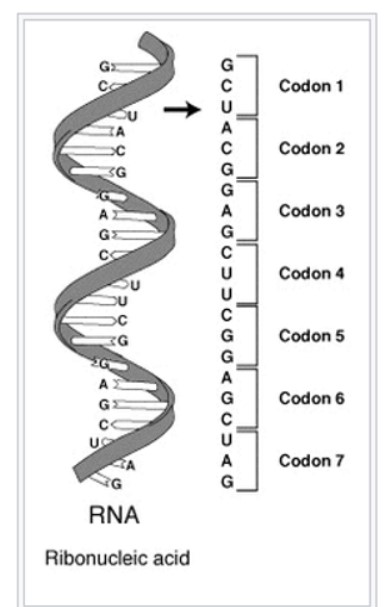
A series of codons in part of a messenger RNA (mRNA) molecule. Each codon consists of three nucleotides, usually corresponding to a single amino acid. The nucleotides are abbreviated with the letters A, U, G, and C. This is mRNA, which uses U (uracil). DNA uses T (thymine) instead. This mRNA molecule will instruct a ribosome to synthesize a protein according to this code. Source
from Bio.Data import CodonTable print(CodonTable.unambiguous_rna_by_name['Standard'])
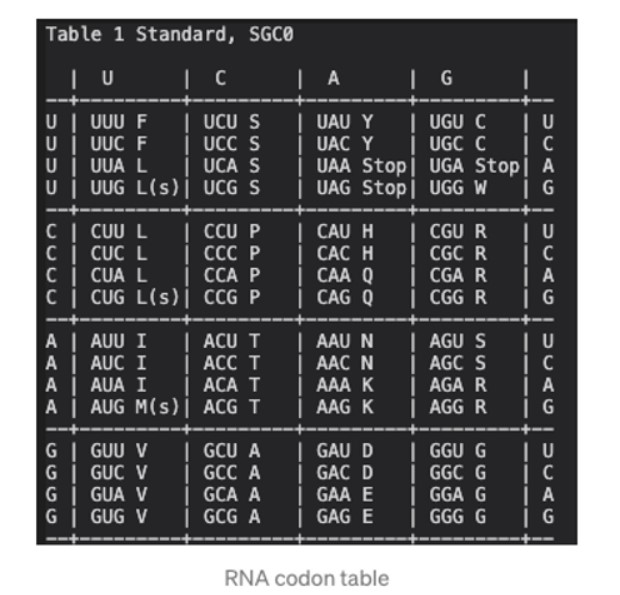
Let’s now identify all the Proteins (chains of amino acids), basically separating at the stop codon, marked by *. Then let’s remove any sequence less than 20 amino acids long, as this is the smallest known functional protein (if curious).
#Identify all the Proteins (chains of amino acids) Proteins = Amino_Acid.split('*') # * is translated stop codon df = pd.DataFrame(Proteins) df.describe() print('Total proteins:', len(df))def conv(item): return len(item)def to_str(item): return str(item)df['sequence_str'] = df[0].apply(to_str) df['length'] = df[0].apply(conv) df.rename(columns={0: "sequence"}, inplace=True) df.head()# Take only longer than 20 functional_proteins = df.loc[df['length'] >= 20] print('Total functional proteins:', len(functional_proteins)) functional_proteins.describe()
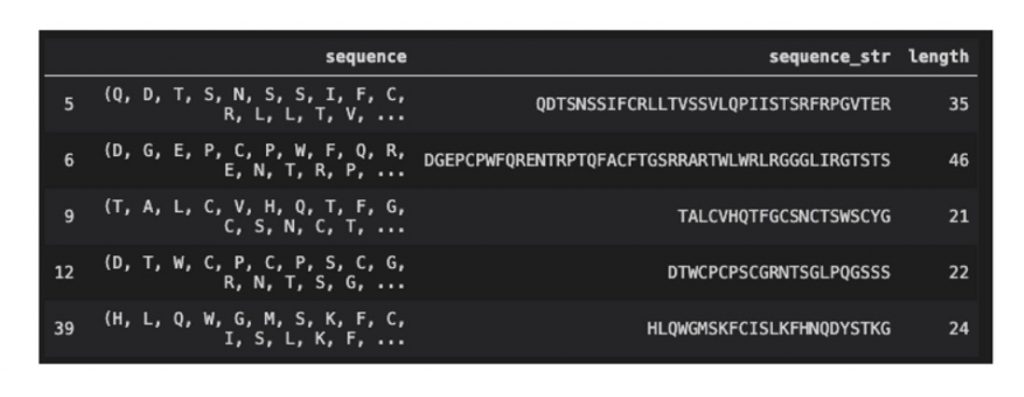
Protein Analysis With The Protparam Module In Biopython using ProtParam.
Available Tools in ProtParam:
- count_amino_acids: Simply count the number times an amino acid is repeated in the protein sequence.
- get_amino_acids_percent: The same as only returns the number in the percentage of the entire sequence.
- molecular_weight: Calculates the molecular weight of a protein.
- aromaticity: Calculates the aromaticity value of a protein according to Lobry & Gautier (1994, Nucleic Acids Res., 22, 3174-3180).
- flexibility: Implementation of the flexibility method of Vihinen et al. (1994, Proteins, 19, 141-149).
- isoelectric_point: This method uses the module IsoelectricPoint to calculate the pI of a protein.
- secondary_structure_fraction: This method returns a list of the fraction of amino acids that tend to be in helix, turn, or sheet.
- Amino acids in Helix: V, I, Y, F, W, L.
- Amino acids in Turn: N, P, G, S.
- Amino acids in Sheet: E, M, A, L.
The list contains 3 values: [Helix, Turn, Sheet].
from __future__ import division poi_list = [] from Bio.SeqUtils import ProtParam for record in Proteins[:]: print("\n") X = ProtParam.ProteinAnalysis(str(record)) POI = X.count_amino_acids() poi_list.append(POI) MW = X.molecular_weight() MW_list.append(MW) print("Protein of Interest = ", POI) print("Amino acids percent = ",str(X.get_amino_acids_percent())) print("Molecular weight = ", MW_list) print("Aromaticity = ", X.aromaticity()) print("Flexibility = ", X.flexibility()) print("Isoelectric point = ", X.isoelectric_point()) print("Secondary structure fraction = ", X.secondary_structure_fraction())
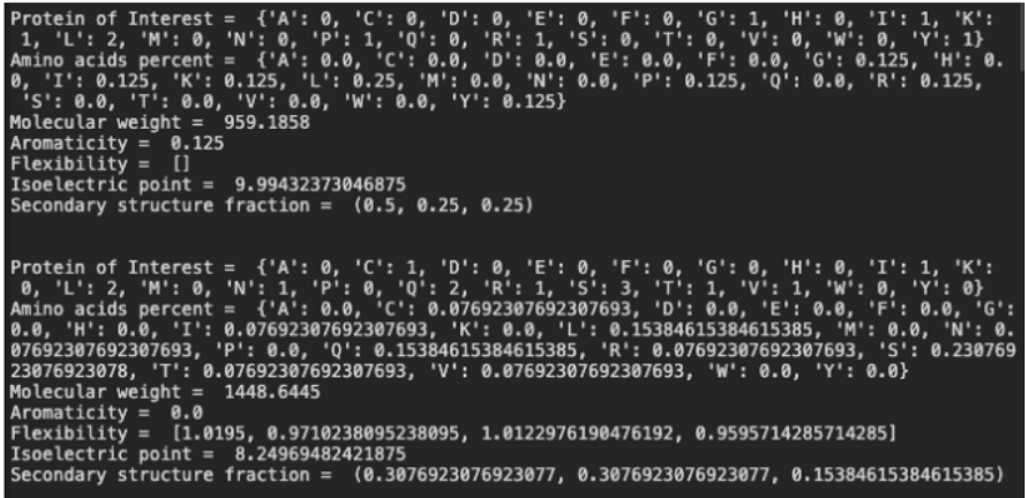
Plot the results:
MoW = pd.DataFrame(data = MW_list,columns = ["Molecular Weights"] )#plot POI poi_list = poi_list[48] plt.figure(figsize=(10,6)); plt.bar(poi_list.keys(), list(poi_list.values()), align='center')
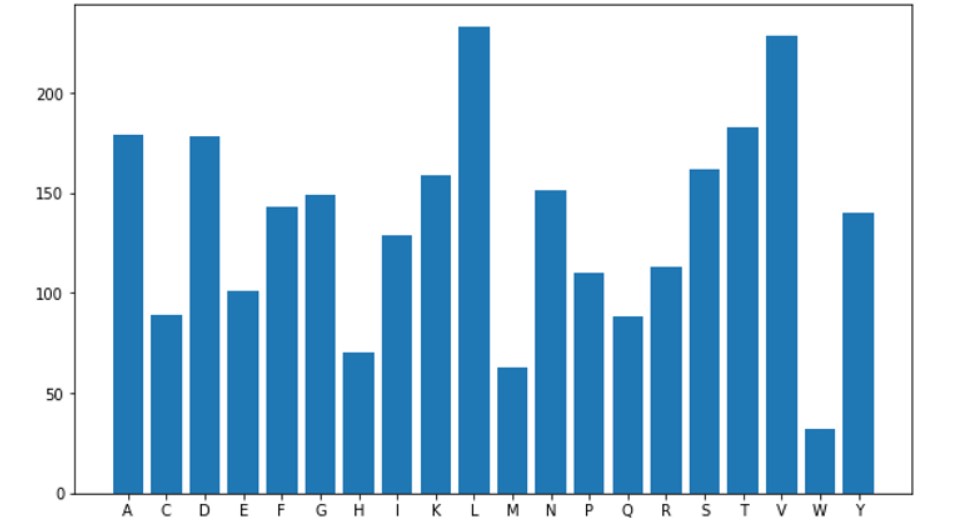
Looks like the number of Lysines(L) and Valines(V) are high in this protein which indicates a good number of Alpha-Helices.
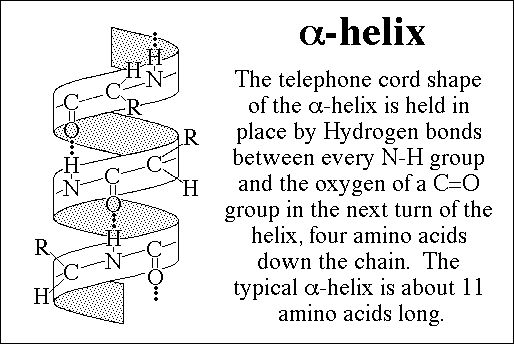
Now let us compare the similarity among COVID-19/COV2, MERS, and SARS.
Load the DNA sequence file (FASTA) each of SARS, MERS, and COVID-19.
#Comparing Human Coronavirus RNA from Bio import pairwise2SARS = SeqIO.read("/coronavirus/sars.fasta", "fasta")MERS = SeqIO.read("/coronavirus/mers.fasta", "fasta")COV2 = SeqIO.read("/coronavirus/cov2.fasta", "fasta")
#Sequence Lengths: SARS: 29751, COV2: 29903, MERS: 30119
Before comparing the similarity let us visualize the DNA each of COV2, SARS, and MERS respectively.
#Execute on terminal Squiggle cov2.fasta sars.fasta mers.fasta --method=gates --separate
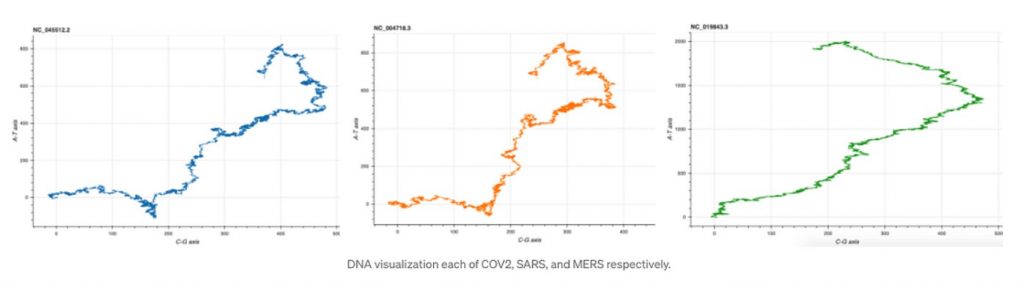
As we can observe the DNA structure of COV2 and SARS are almost identical, while that of MERS is a little different than the two.
Now let us use the Sequence alignment technique to compare the similarity among all the DNA sequences.
Sequence alignment is the process of arranging two or more sequences (of DNA, RNA, or protein sequences) in a specific order to identify the region of similarity between them.
Identifying the similar region enables us to infer a lot of information like what traits are conserved between species, how close different species genetically are, how species evolve, etc.
Pairwise sequence alignment compares only two sequences at a time and provides the best possible sequence alignments. Pairwise is easy to understand and exceptional to infer from the resulting sequence alignment.
Biopython provides a special module, Bio.pairwise2 to identify the alignment sequence using the pairwise method. Biopython applies the best algorithm to find the alignment sequence and it is par with other software.
# Alignments using pairwise2 alghoritm SARS_COV = pairwise2.align.globalxx(SARS.seq, COV2.seq, one_alignment_only=True, score_only=True) print('SARS/COV Similarity (%):', SARS_COV / len(SARS.seq) * 100) MERS_COV = pairwise2.align.globalxx(MERS.seq, COV2.seq, one_alignment_only=True, score_only=True) print('MERS/COV Similarity (%):', MERS_COV / len(MERS.seq) * 100) MERS_SARS = pairwise2.align.globalxx(MERS.seq, SARS.seq, one_alignment_only=True, score_only=True) print('MERS/SARS Similarity (%):', MERS_SARS / len(SARS.seq) * 100)
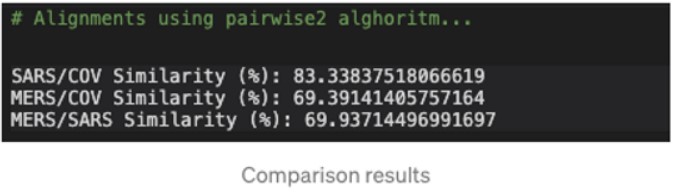
Phylogenetic analysis of the complete viral genome (29,903 nucleotides) revealed that the COVID-19 virus was most closely related (83.3% nucleotide similarity) to a group of SARS-like coronaviruses (genus Betacoronavirus, subgenus Sarbecovirus) that had previously been found in bats in China.
Plot the results:
# Plot the data X = ['SARS/COV2', 'MERS/COV2', 'MERS/SARS'] Y = [SARS_COV/ len(SARS.seq) * 100, MERS_COV/ len(MERS.seq)*100, MERS_SARS/len(SARS.seq)*100] plt.title('Sequence identity (%)') plt.bar(X,Y)
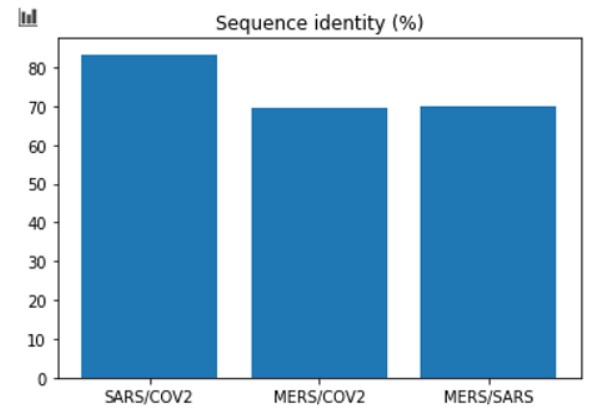
You can get the code here in this GitHub repo.
Conclusion
So we have seen how we can interpret, analyze the COVID-19 DNA sequence data, and tried to get as many insights regarding the proteins that made it up. In our result, we got the number of Leucine(L) and Valines(V) high in this protein which indicates a good number of Alpha-Helices.
Later we compared the COVID-19 DNA with MERS and SARS. And we saw that COVID-19 is closely related to SARS.
That is all for this article. I hope you guys have enjoyed reading it, please share your suggestions/views/questions in the comment section.
Thanks for Reading !!!
Note: This article was originally published on theaidream, and kindly contributed to AI Planet (formerly DPhi) to spread the knowledge.