T5 and GPT 2
T5 - Text-To-Text Transfer Transformer
T5 is a model architecture introduced in the paper "Exploring the Limits of Transfer Learning with a Unified Text-to-Text Transformer" by Google Research Team: Colin Raffel, Noam Shazeer, Adam Roberts, Katherine Lee, Sharan Narang, Michael Matena, Yanqi Zhou, Wei Li, Peter J. Liu. T5 is designed as a general-purpose framework for various natural language processing tasks, casting all tasks into a unified text-to-text format.
Abstract
Transfer learning, where a model is first pre-trained on a data-rich task before being finetuned on a downstream task, has emerged as a powerful technique in natural language processing (NLP). The effectiveness of transfer learning has given rise to a diversity of approaches, methodology, and practice. In this paper, we explore the landscape of transfer learning techniques for NLP by introducing a unified framework that converts all text-based language problems into a text-to-text format. Our systematic study compares pre-training objectives, architectures, unlabeled data sets, transfer approaches, and other factors on dozens of language understanding tasks. By combining the insights from our exploration with scale and our new “Colossal Clean Crawled Corpus”, we achieve state-of-the-art results on many benchmarks covering summarization, question answering, text classification, and more. To facilitate future work on transfer learning for NLP, we release our data set, pre-trained models, and code.
How T5 works?

T5 introduces a paradigm where all NLP tasks are treated as a text-to-text problem, meaning both inputs and outputs are treated as sequences of text. For instance, for text classification, the input might be the text of a document and the output might be a label or category. For translation, the input could be a sentence in one language, and the output would be the corresponding sentence in another language.
The T5 model architecture is based on the transformer architecture, similar to BERT and RoBERTa. It involves an encoder-decoder structure. The encoder takes the input text and transforms it into a fixed-size vector representation. The decoder then takes this vector and generates the output text. The key innovation in T5 is its formulation of various tasks into this text-to-text format, allowing the use of the same architecture for different tasks.
The full details of the investigation can be found in T5 paper, including experiments on:
- Model architectures: They observed Encoder-decoder models generally outperformed "decoder-only" language models.
- Pre-training objectives: Where it confirmed that fill-in-the-blank-style denoising objectives (where the model is trained to recover missing words in the input) worked best and that the most important factor was the computational cost.
- Unlabeled datasets: Which demonstates that training on in-domain data can be beneficial but that pre---training on smaller datasets can lead to detrimental overfitting.
- Training strategies: The multitask learning could be close to competitive with a pre-train-then-fine-tune approach but requires carefully choosing how often the model is trained on each task.
- Scale: Scaling up the model size, the training time, and the number of ensembled models to determine how to make the best use of fixed compute power.
GPT2 - Text-To-Text Transfer Transformer
GPT-2, or Generative Pre-trained Transformer 2, is a model developed by OpenAI and represents the second iteration of the GPT series. It was introduced in the paper "Language Models are Unsupervised Multitask Learners" by Alec Radford, Jeffrey Wu, Rewon Child, David Luan, Dario Amodei, Ilya Sutskever.
Abstract
Natural language processing tasks, such as question answering, machine translation, reading comprehension, and summarization, are typically
approached with supervised learning on taskspecific datasets. We demonstrate that language
models begin to learn these tasks without any explicit supervision when trained on a new dataset
of millions of webpages called WebText. When
conditioned on a document plus questions, the answers generated by the language model reach 55
F1 on the CoQA dataset - matching or exceeding
the performance of 3 out of 4 baseline systems
without using the 127,000+ training examples.
The capacity of the language model is essential
to the success of zero-shot task transfer and increasing it improves performance in a log-linear
fashion across tasks. Our largest model, GPT-2,
is a 1.5B parameter Transformer that achieves
state of the art results on 7 out of 8 tested language modeling datasets in a zero-shot setting
but still underfits WebText. Samples from the
model reflect these improvements and contain coherent paragraphs of text. These findings suggest
a promising path towards building language processing systems which learn to perform tasks from
their naturally occurring demonstrations.
How GPT-2 Architecture works?
GPT-2 is based on the transformer architecture and is a generative language model. Unlike models like BERT that are designed for bidirectional understanding, GPT-2 is autoregressive, meaning it generates one token at a time based on the tokens that came before it. This makes it well-suited for tasks like text generation, completion, and summarization.
The model is pre-trained on a massive corpus of text data and learns to predict the next word in a sentence. This process equips it with a strong understanding of grammar, context, and even some level of reasoning. The "transformer decoder" architecture, used in GPT-2, allows it to consider both previous words and learned context when generating text.
GPT-2 Architecture
- Comprises stacked decoder blocks from the transformer architecture.
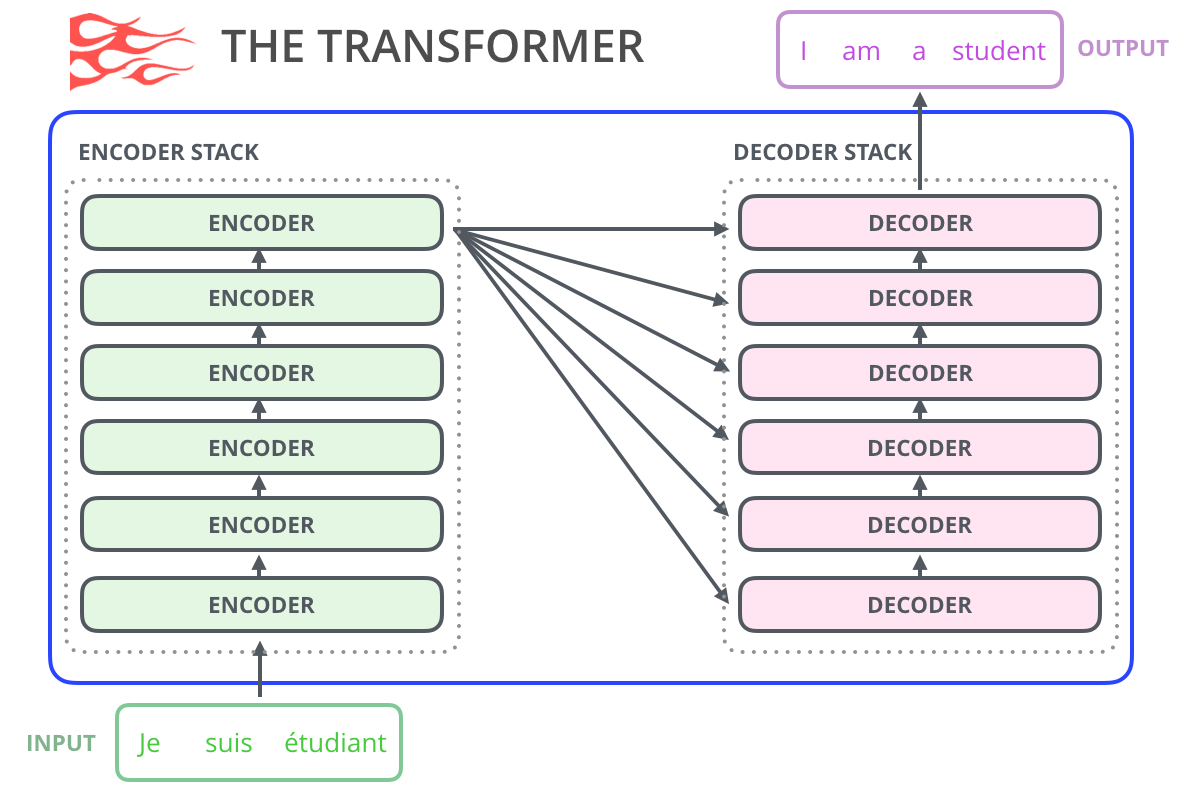
- Differs from standard transformers in context vector initialization and self-attention.
Context Handling
- GPT-2 initializes context vector with zeros for the first word embedding.
- The word vectors used for the first layer of GPT-2 are not simple one-hot tokenizations but byte pair encodings. The byte pair encoding scheme compresses an (arbitrarily large?) tokenized word list into a set volcabulary size by recursively keying the most common word components to unique values (e.g. 'ab'=010010, 'sm'=100101, 'qu'=111100, etecetera).
- In GPT-2 masked self-attention is used instead: the decoder is only allowed (via obfuscation masking of the remaining word positions) to glean information from the prior words in the sentence (plus the word itself).
Training Data
- Trained on a substantial web scrape dataset, resembling industrial-scale collection.
- Standard transformer approach with batch size of 512, defined sentence length, 50,000-word vocabulary.
- During evaluation, adapts to one-word-at-a-time input by storing past context vectors.
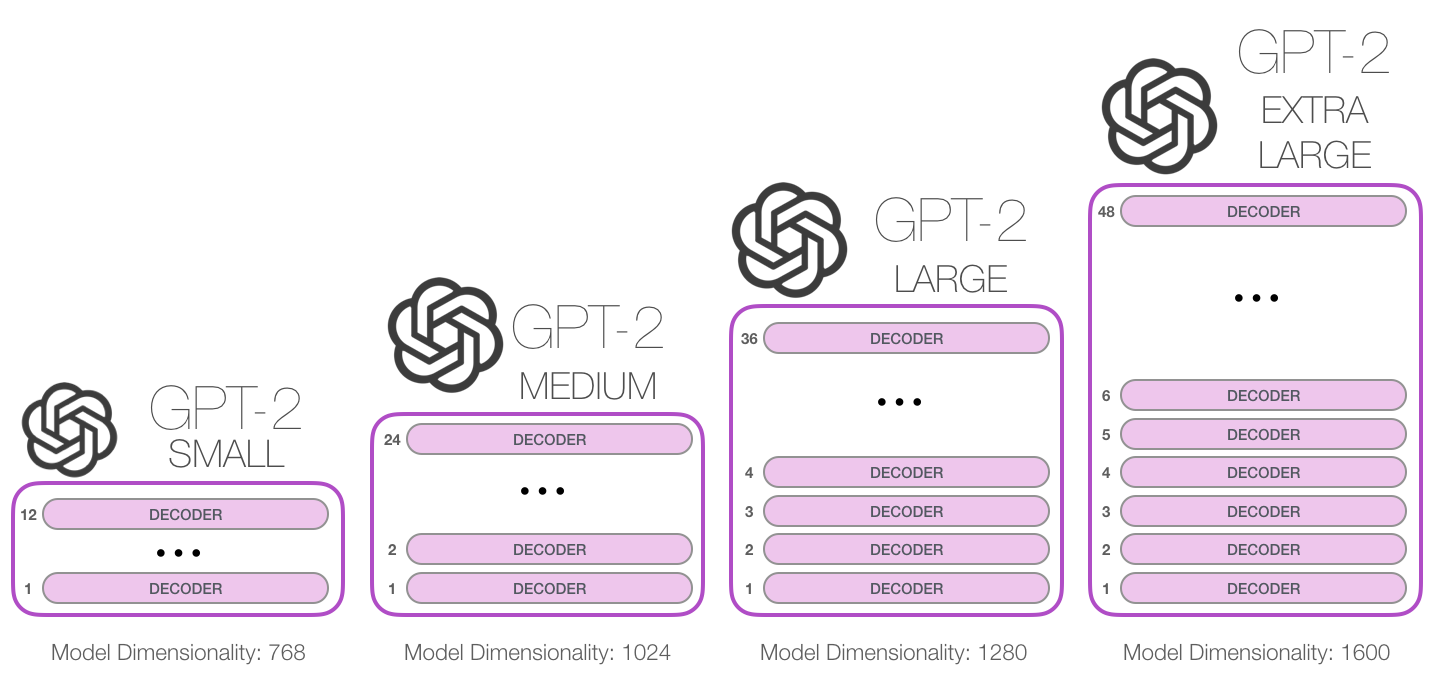
The GPT2, and some later models like TransformerXL and XLNet are auto-regressive in nature. BERT is not. That is a trade off. In losing auto-regression, BERT gained the ability to incorporate the context on both sides of a word to gain better results.
Reference:
- Google AI Blog on T5
- The Illustrated GPT-2 by Jay Alammar
- Research papers: